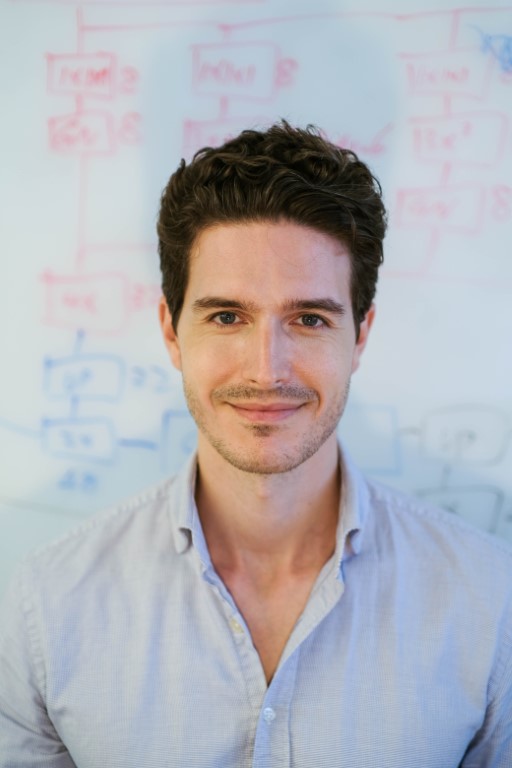
I'm Assistant Professor at Macquarie University in Sydney, Australia. I'm also an upcoming AI2050 Fellow, Australian Research Council DECRA Fellow, and ARC Discovery Project Principal Investigator.
Previously, I was a Presidential Scholar at Columbia University and completed my PhD at the University of Oxford.
My research focuses on understanding modern artificial neural networks, such as large language models, through theoretical analysis, behavioral evaluation, and interpretability methods. Drawing on philosophy and cognitive science, I aim to establish frameworks for fair and meaningful comparisons between machines and humans in domains such as language processing and reasoning. In turn, I use insights from studying neural networks to inform theories of human cognition.